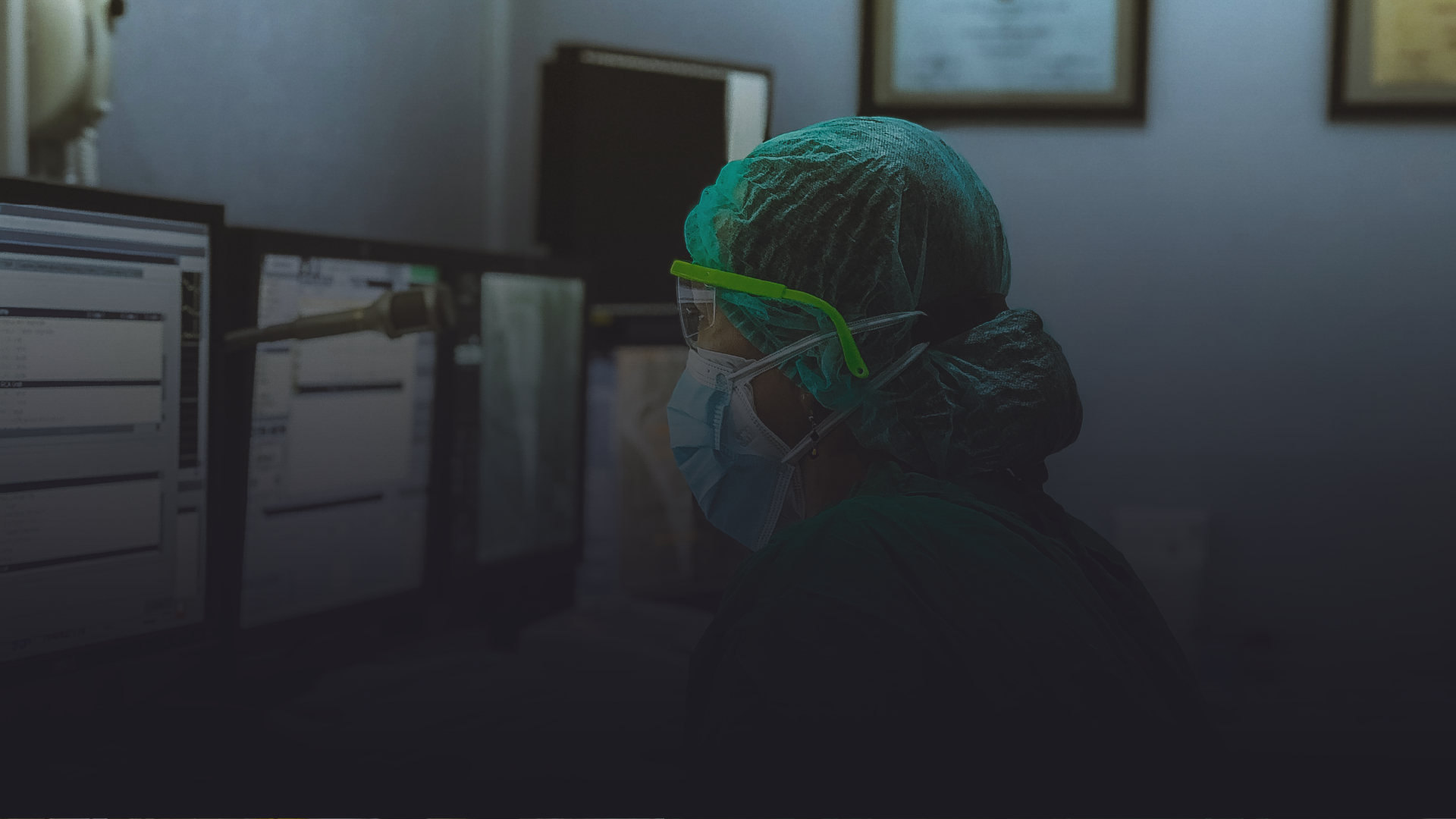
Medical Documentation
Medical Vocabulary Understood by AI / Case
In today's fast-evolving technological landscape, the integration of AI into healthcare and business documentation is changing how companies manage data and communicate effectively. AAI Labs is currently undertaking a project to create two medicine-related large language models.
One model is designed for general communication and document analysis, while the other specifically targets medical documentation using speech recognition. Vilnius University Hospital Santaros Klinikos (VULSK) is the main testing site, providing specialised audio recordings for model training.
This collaboration ensures the LLM models are highly accurate and applicable in real-world healthcare settings, addressing inefficiencies in data management while enhancing productivity in both healthcare and business sectors.
Objectives
Documentation and communication model
Will scan, analyze, and summarize documents, helping businesses improve communication and decision-making.
The solution will offer insights and predict financial performance based on document content, helping organizations manage resources more efficiently.
Medical speech recognition model
Designed to automate medical documentation, the model listens to doctor-patient conversations, transcribes them, and generates summaries.
The model will ensure accuracy in capturing medical terminology, reducing the need for manual data entry by healthcare professionals, thereby allowing them to focus more on patient care.
Data collection
A key component of this project is the data collection process, which is being carried out by doctors at VULSK . The medical professionals are collecting specialized audio recordings from real doctor-patient conversations, allowing the AI model to train with real-world, domain-specific language. This is crucial for ensuring that the AI can accurately capture the nuances of medical vocabulary and terminology, which often differ significantly from everyday language.
Timeline
The data collection phase is currently underway, with the doctors at VULSK expected to gather the dataset over the next several months. Model training and iterative testing are scheduled to follow, with preliminary results anticipated in the first quarter of 2025. The models will undergo final testing and validation in real-world healthcare settings, with full deployment expected by mid-2026.
Challenges and solutions
Data complexity in business and healthcare
One of the key challenges is managing diverse data formats in administrative and healthcare environments. Documents such as contracts, invoices, and communications from various platforms must be processed accurately. Our model is trained to scan, interpret, and answer queries related to these documents, ensuring the highest level of security by keeping all data within the company infrastructure.
In healthcare, capturing accurate audio data from doctor-patient conversations and processing medical terminology is challenging. Our team addresses this by refining the model’s ability to recognize medical terms, with testing focused on maintaining high accuracy even when audio recordings are lower quality.
Technological integration
By using LLaMA 7b/13b/70b models combined with speech recognition technologies like wav2vec 2.0, Whisper, and AssemblyAI, we ensure smooth and reliable operation across different applications. Moreover, we have set extensive testing phases to refine how the models handle real-world use cases in both sectors.
Expected impact and results
Our model is expected to reduce the time spent on document analysis by up to 50%, increasing overall productivity by automating data extraction and report generation. The model’s high level of document categorization accuracy (over 90%) will allow to optimize workflows, minimize human error, and make informed decisions faster. The medical documentation model will reduce the administrative work for medical personnel, freeing up more time for patient care. By automatically generating accurate summaries of medical interactions, this system can cut documentation time in half, achieving up to 95% accuracy in medical data transcription.
Future prospects
One of the most exciting aspects of this project is its focus on Lithuanian, a low-resource language, which makes the development of these AI models innovative. Lithuanian, like many other less widely spoken languages, lacks large datasets and extensive language processing resources. This project not only addresses the needs of Lithuanian medical professionals but also opens the door for similar initiatives in other countries that face challenges with low-resource languages.
The long-term vision for the project is to replicate and expand this model to other low-resource languages, improving healthcare sector efficiency in various countries. By cloning the system for different languages, we aim to enable other healthcare systems to adopt similar solutions, improving patient care and administrative efficiency.
Client
Vilnius University Hospital Santaros klinikos
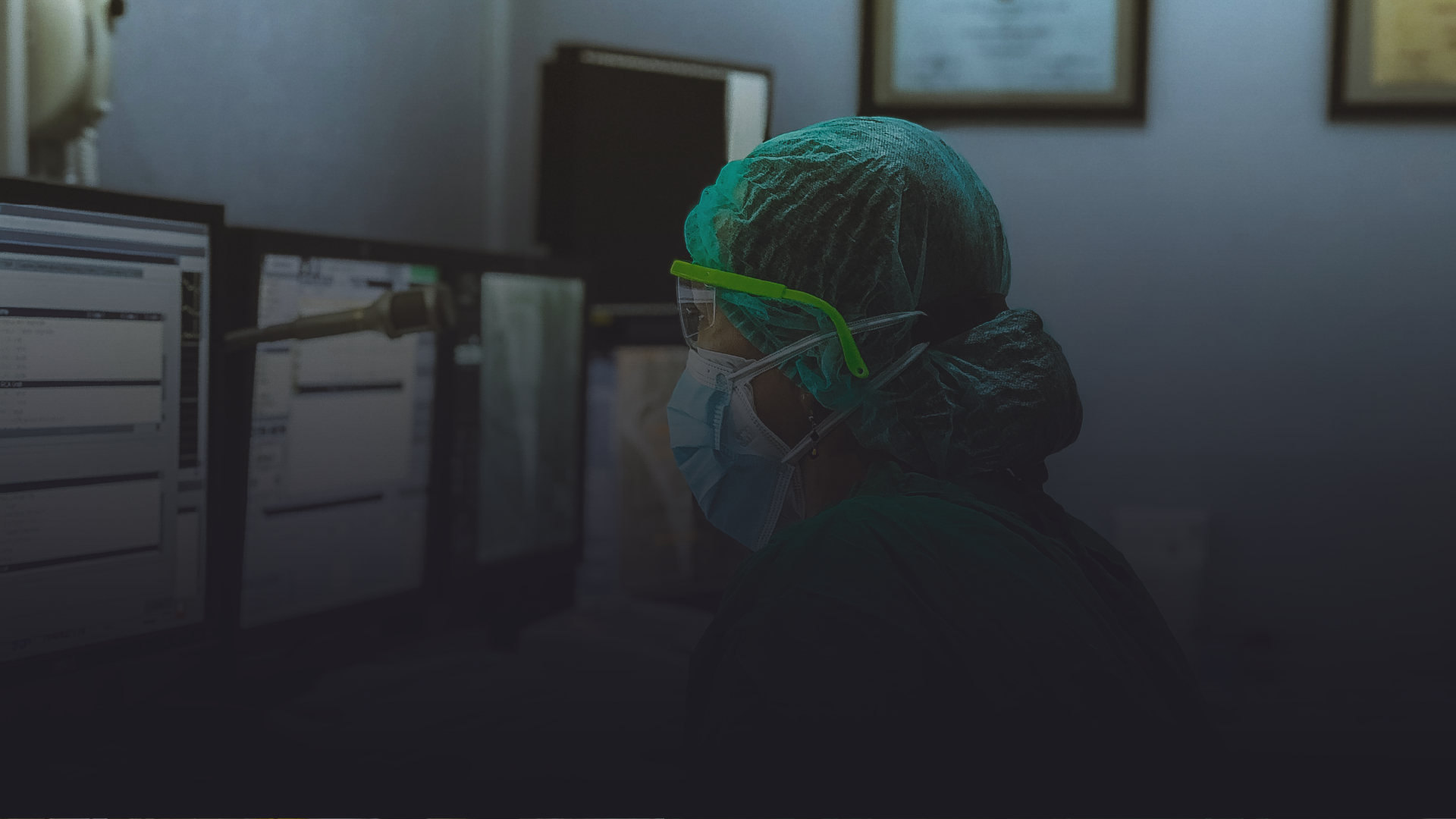