
Plagiarism & Fraud Detection
Agriculture Application Evaluation / Case
AAI Labs has started developing an automated system for the National Paying Agency (NMA) under the Ministry of Agriculture of the Republic of Lithuania for analyzing funding requests, to reduce the risks of duplicate financing and fraudulent submissions.
NMA receives over 8,000 applications annually, creating a significant challenge for manual review and fraud detection. Current approach is time-consuming and labor-intensive, with risks of errors, especially in detecting organized fraud schemes. Also, the data exists in various unstructured formats, complicating analysis.
Core features of the solution
The system processes funding applications in multiple formats, converting them into structured data for analysis. The data extraction module handles JSON, PDF, Word, and others, using Optical Character Recognition (OCR) where necessary.
Advanced algorithms analyze the structure, vocabulary, and style of writing. The engine utilizes BERT-based NLP models to compare text for similarities and patterns.
A machine learning model, trained on historical data of fraudulent projects, then identifies risky applications. Key fraud indicators include unusual financial patterns and project inconsistencies. The system assigns a risk score to each application based on historical fraud cases and anomaly detection techniques.
The tool generates a standard profile for each project, categorizing applications based on key parameters such as required funding amount, project type, and relevant performance indicators.
Detailed reports provide an overview of application similarities, including a risk profile and analysis of potentially fraudulent or artificially generated submissions. These reports also visualize risk data through interactive dashboards.
All data is processed and stored within the client’s internal infrastructure; no external services are used. Sensitive information is encrypted both during transmission and storage using AES-256 encryption standards. Role-based access Control ensures that only authorized personnel have access to confidential data.
System architecture
The system is built using a modular and scalable architecture designed for high performance and security. It is structured into several key layers, each responsible for different aspects of the data processing workflow. The input layer handles the uploading of data files and performs initial pre-processing tasks, including tokenization and OCR to convert these documents into structured formats suitable for analysis. Once the data is prepared, the processing layer applies advanced machine learning models for text comparison, plagiarism detection, and fraud identification. Finally, the output layer generates comprehensive reports that summarize the system’s findings.
Future prospects
The potential of this solution to evolve into a more comprehensive tool that can be applied to other public sector domains is evident. By continuously improving through machine learning retraining and model optimization, the system can increase its accuracy and detection capabilities. Furthermore, the scalability of the architecture opens up opportunities for international or multi-agency use.
Client
Nacionalinė mokėjimo agentūra (NMA), Ministry of Agriculture of the Republic of Lithuania
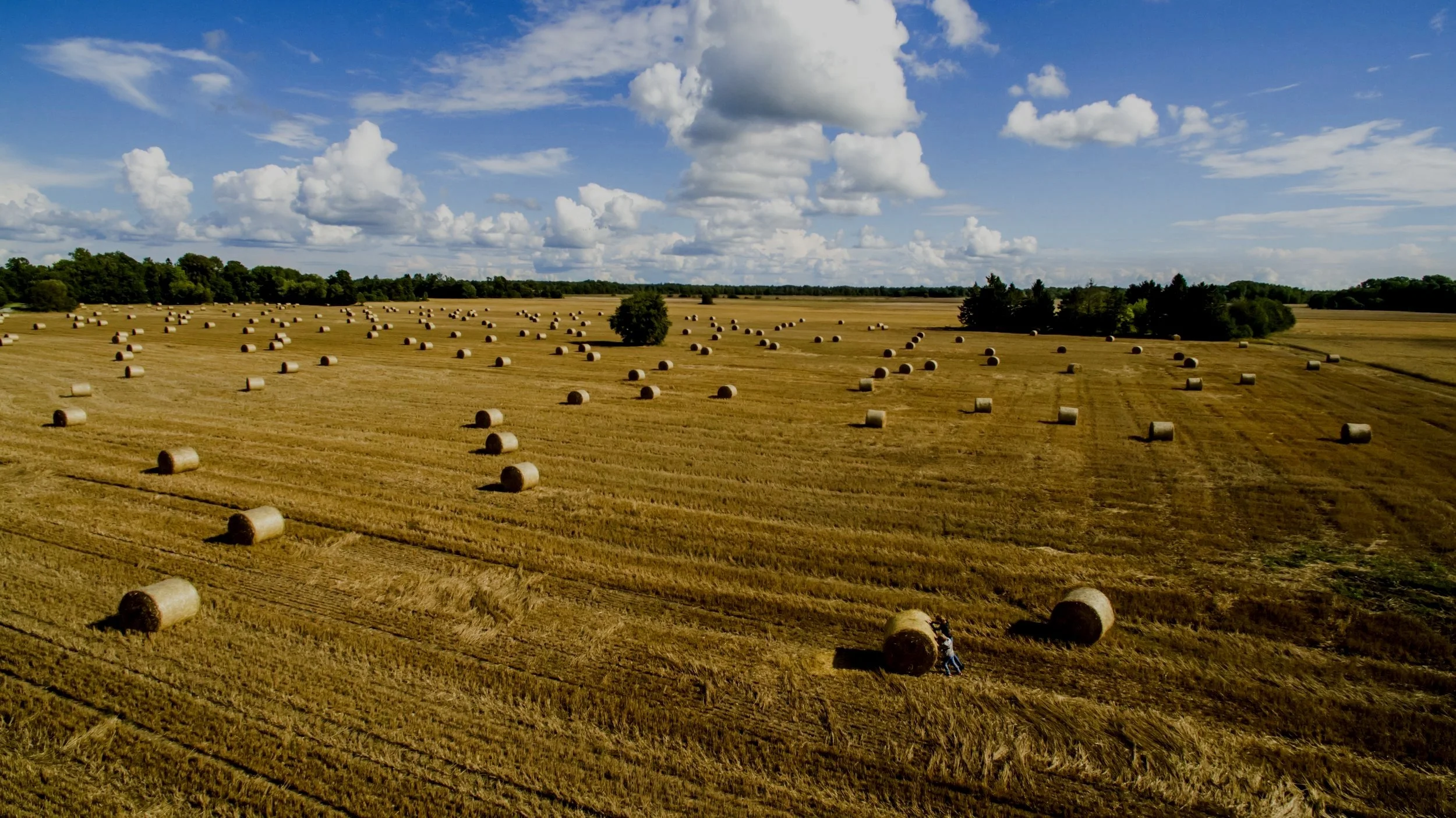