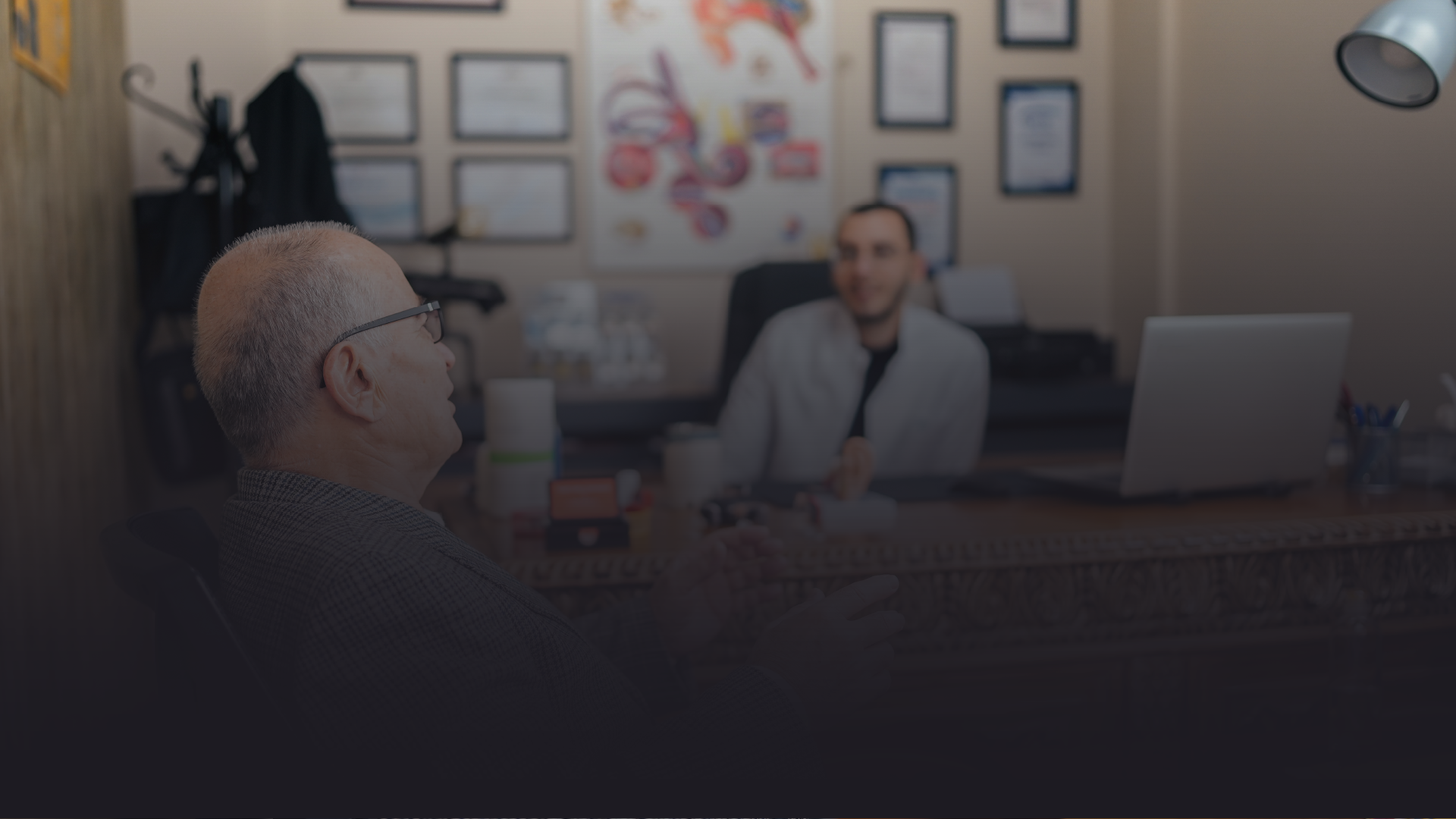
Call Center Automation
Speech Recognition in Medical Centers / Case →
AAI Labs is developing an AI-driven call center assistant prototype “Medicall” to streamline call management and improve patient experience.
The main medical center in Kaunas receives over 95,000 calls every month across 34 operators. Patients encounter long waiting times and delayed responses due to the overwhelming number of calls and the complex nature of patient inquiries. AAI Labs is developing an AI-driven call center assistant prototype “Medicall” to streamline call management and improve patient experience.
Objectives
The main goal of this project is to change patient inquiry management, appointment scheduling, and communication. By automating routine tasks and providing real-time assistance to operators, the solution will transform the call center into a more efficient, responsive system, ensuring a better experience for patients and healthcare providers alike. Automated speech-to-text transcription will convert live patient conversations into real-time text, ensuring operators can accurately understand and respond to inquiries. Contextual response generation will suggest real-time responses based on patient queries, helping operators answer questions faster and more accurately. Appointment scheduling will handle appointment bookings, cross-referencing doctor availability and patient preferences for efficient scheduling. Summary generation will automatically generate a call summary after each call for the operator to review and finalize, improving documentation speed and accuracy.
Solution
At the core of this innovation is the use of bespoke and powerful AI models, including Whisper and Wav2Vec2, which AAI Labs had designed to transcribe conversations in Lithuanian language in real time, even in noisy or challenging environments. These models will enable the system to capture patient conversations with at least 85% accuracy and transcribe them into text that operators can use for quick and precise decision-making. Reinforcement learning algorithms will enhance the system’s ability to understand context and generate relevant responses reducing the time spent answering routine questions.
In addition to speech recognition, the system will be scalable and have the ability to integrate with existing softwares, including patient booking platforms and electronic health records. By automating appointment scheduling, the system will handle bookings with precision and reduce the possibility of errors. Additionally, the solution will generate automated summaries of calls, allowing operators to finalize documentation quickly and move on to the next call without delays.
The solution is designed with a user-friendly interface in mind, providing easy access to real-time transcriptions, suggested responses, and appointment management tools, to reduce the cognitive load on operators. This simplified workflow will help operators to handle a higher volume of calls with greater accuracy and lead to better service for patients.
Implementation plan
During the first stage we will gather and analyze a dataset of 50,000 patient interactions and call transcripts, which will be used to train the model. This step will ensure that the system is familiar with the healthcare terminology and patient communication.
Following this, the testing in a controlled environment will be conducted to ensure system’s accuracy and performance before deployment. This phase will focus on fine-tuning the transcription accuracy, ensuring that medical terms are correctly identified, and optimizing the real-time response generation capabilities. Once these tests are successfully completed, the system will be integrated with existing infrastructure, including the booking and health records platforms.
Future prospects
After successful deployment of the system, AAI Labs solution can be easily adapted to other healthcare institutions facing similar operational challenges. The system’s scalability will allow for quick deployment in clinics and hospitals across Lithuania. Looking further ahead, as the solution evolves, it can be customized for other low-resource languages beyond Lithuanian, allowing it to be implemented in international healthcare settings where similar challenges exist.
Client
Kauno miesto poliklinika
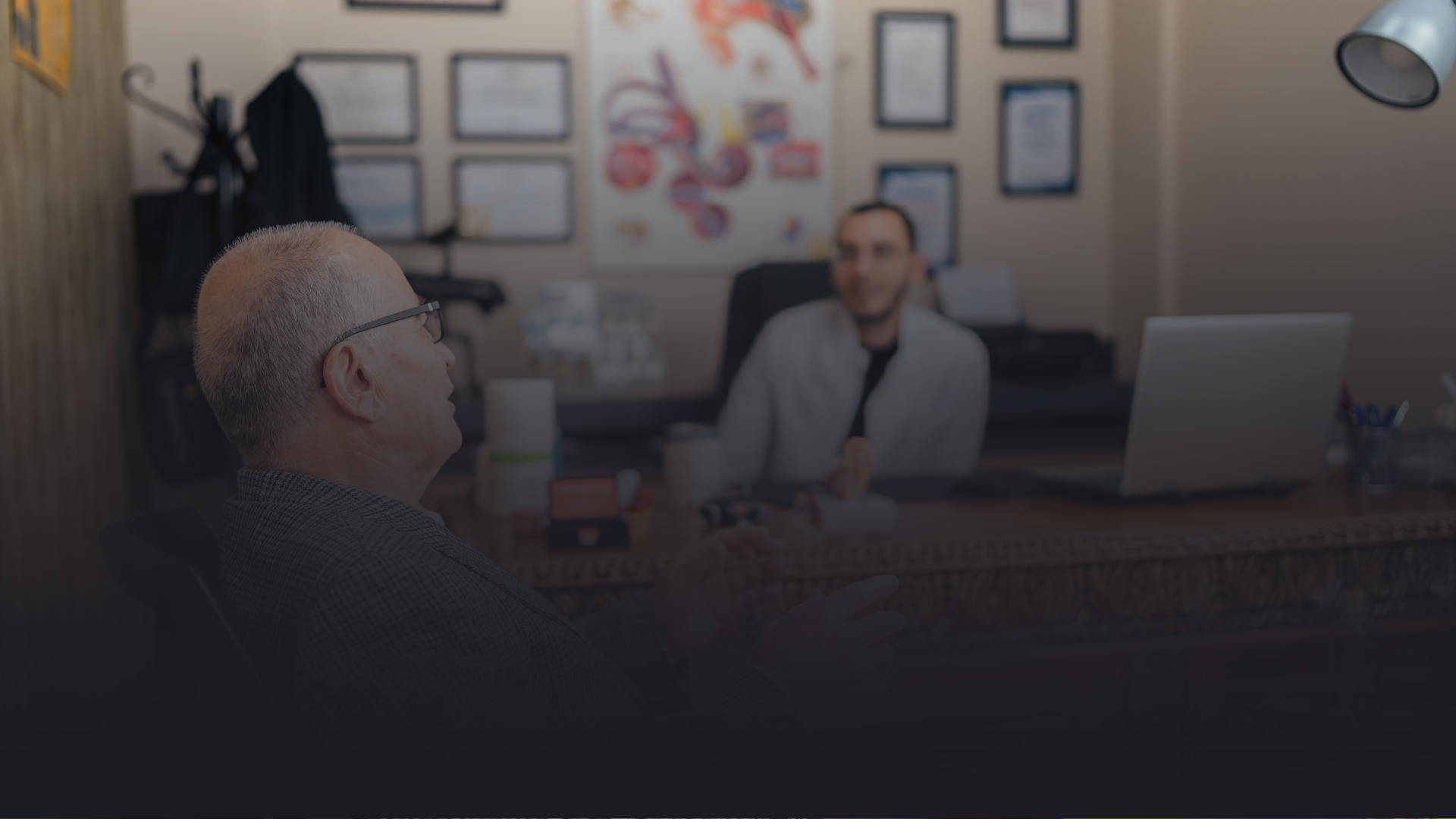